How AI is Transforming Conservation
Wildlife and biodiversity may not be the first things that come to mind when you think of AI, but conservation organizations like WWF have long used a variety of technologies to monitor and manage the health of wildlife and ecosystems.
New AI and machine learning applications are rapidly accelerating and increasing the scale and effectiveness of conservation efforts worldwide.
Today, we face a triple challenge with food and water systems, climate change, and biodiversity and species loss. Each of these is complex and occurs on a massive scale—precisely the type of challenge that AI excels at addressing.
In complex situations involving biodiversity conservation, disaster resilience, public health or combatting illegal wildlife trade, when there are massive amounts of data, limited resources and other constraints, AI models can analyze faster, more cost-effectively and much more efficiently than teams of humans could.
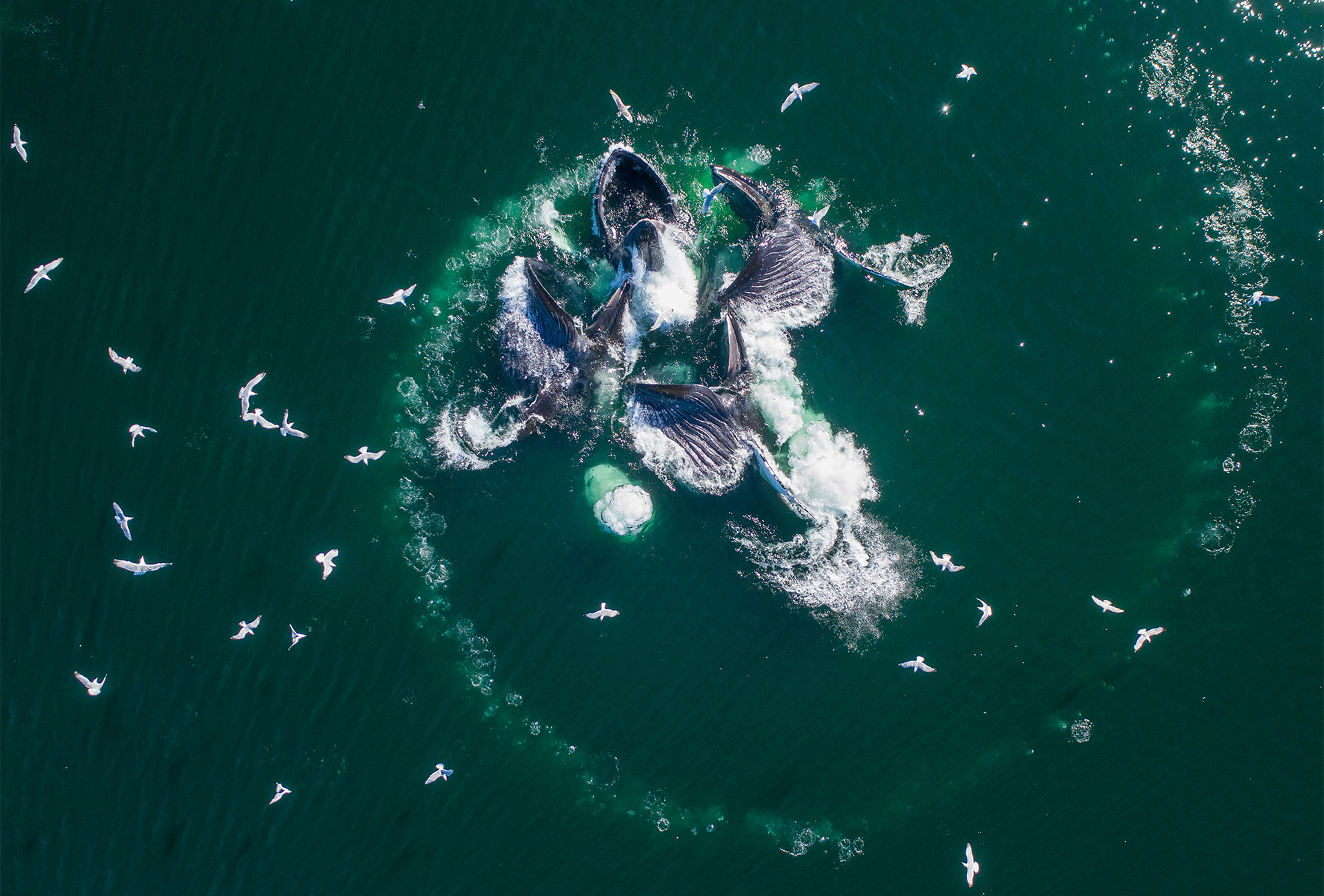
Drone footage of humpback whales bubble-net feeding in Frederick Sound, Alaska.
What is AI for Conservation?
Artificial intelligence (AI) enables computer technologies to perform advanced functions, including the ability to see, understand and translate spoken and written language, analyze data, and make recommendations.
Machine learning is a subset of artificial intelligence (as are deep learning, robotics, and natural language processing) that enables systems to learn and improve from experience. Machine learning uses algorithms to analyze large amounts of data, learn from insights, and make informed decisions. The more (and more representative) data used, the more accurate and useful the model will be.
In a WWF webinar on artificial intelligence and conservation, Bistra Dilkina, Associate Professor of Computer Science at the University of Southern California, shares that “in conservation, AI and machine learning are primarily used to answer two different types of questions: predictive (“What should we expect?”) and decision making (“What should we do?”).”
In a recent example of predictive AI for conservation, WWF-Netherlands collaborated with computer scientists and artificial intelligence experts to develop an advanced computer model to combat deforestation. Piloted in Borneo and Gabon, the tool can predict forest loss up to six months in advance with 80% accuracy, answering “What can we expect?” and helping local populations prepare or even prevent that loss.
In response to concerns, “Where should we place cameras, plan wildlife corridors or send ranger patrols?” AI models can provide powerful decision support to inform strategy and policy to protect vulnerable species and ecosystems. AI models assist researchers and rangers in protecting species as diverse as humpback whales, koalas and snow leopards, supporting tasks ranging from anti-poaching patrols to monitoring species.
The potential for using AI in conservation is enormous. Over 20,000 species on the International Union for Conservation of Nature’s (IUCN) Red List of Threatened Species are labeled as “data deficient,” meaning there’s not enough information to properly assess their risk of extinction. The use of AI and machine learning could reduce that number significantly.
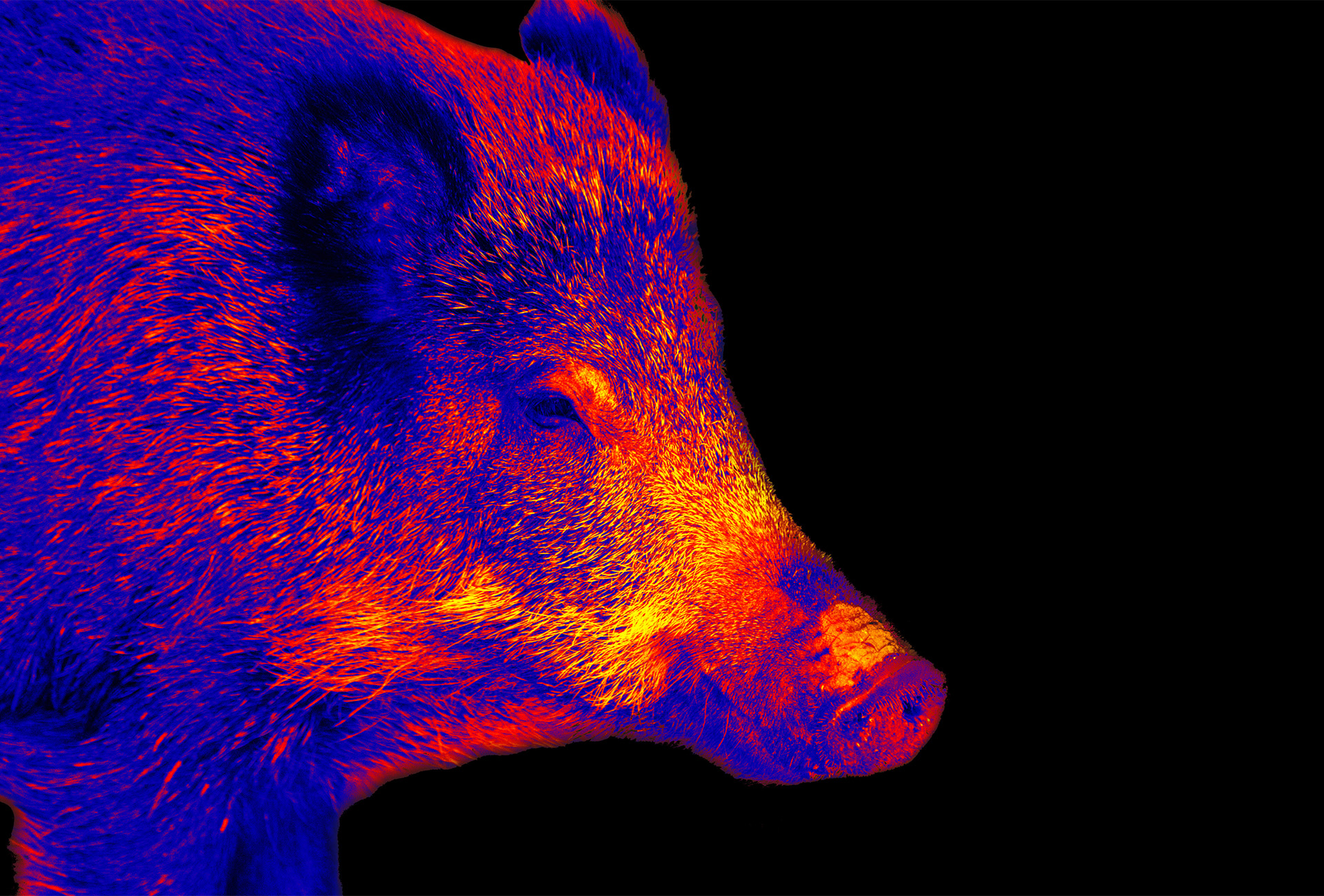
This wild boar was spotted using a thermal imaging camera. Thermal imaging drone surveys are becoming increasingly popular as they can help identify more elusive animals.
How is AI used in conservation?
Multiple trends are converging, resulting in a dramatic increase in AI applications for conservation:
First, technologies to gather conservation data in the field are improving and increasing, resulting in a massive amount of raw data. Here are a handful of examples:
-
Camera Traps
Over 1 million camera traps are now deployed around the world. Camera traps are among conservation’s most widely deployed technologies, using infrared sensors to detect an animal crossing their path and trigger the digital photograph or video. The data collected from camera traps, often operating remotely for months, provides crucial details on a species’ location, population, and behavior, helping conservationists understand when, where, and how to protect them.
Camera trap data can be used in a number of ways. Wildlife Protection Solutions, based in Colorado, uses custom camera traps and 24/7 monitoring in wildlife reserves around the world to fight wildlife crime in real time with AI systems that detect wildlife threats, intrusions and illegal activities.
Landscape connectivity and wildlife corridor conservation planning can be essential for species survival. The open-source platform LINC (Lion Identification Network of Collaborators) is a good example of how machine learning can be applied to African wildlife conservation.
The African lion has lost 42% of its habitat since the turn of the millennium, and populations have become increasingly isolated from each other. Data on the lions’ migratory behavior can help maintain genetic diversity and prevent populations from dying out due to inbreeding. Data collected via LINC will provide insights into the areas where the lions spend most of their time and when and how they migrate.
The LINC Lion identification algorithms use two methods to match individual lions:
The first uses the lion’s whisker patterns, comparing them between individuals. The second method looks at the feature groups of individual lion faces, akin to how we identify faces. Both AI methodologies allow researchers to process previously unmanageably large datasets. The LINC project is an open-source model allowing a sustained development that benefits the whole African wildlife conservation community.
-
Bioacoustic sensors
WWF-Canada has partnered with the Gitga’at First Nation and North Coast Cetacean Society to launch a multi-year monitoring project called SWAG (Ships, Whales, Acoustics in Gitga’at Territory). SWAG’s four hydrophones collect acoustic data from which researchers can pick out whales, triangulate their precise location, determine their numbers, and track their movement in the Squally Channel.
AI is being used to develop algorithms that can differentiate between orcas, fin whales and humpback whales. By comparing incoming acoustic data against an existing audio library of whale sounds, software learns how to identify individual species and understand the nature of the vocalization—distinguishing between feeding, socializing and other types of calls.
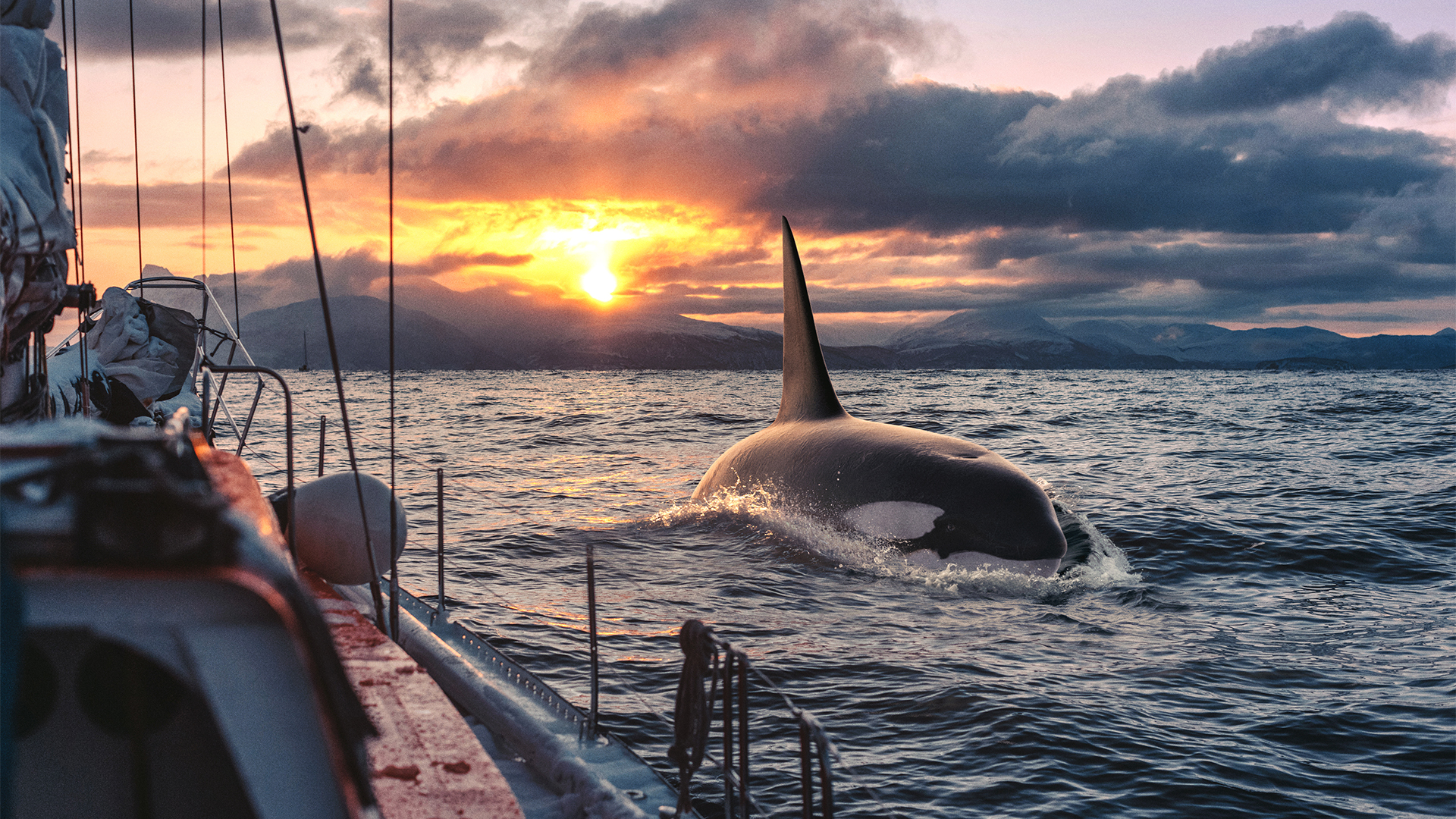
Orca (killer) whale
With this data, SWAG will help the Gitga’at Nation, government agencies and industry implement effective conservation measures to protect whale populations. For example, ships could be alerted when approaching whales or restricted from entering certain areas.
-
Miniaturization of mobile (on-animal) sensors
The Okavango Delta in Botswana represents one of the last strongholds for the endangered African wild dog and through dispersing individuals, the resident population likely acts as a source population for the natural re-colonization of the surrounding regions. Under these circumstances, understanding how and where wild dogs disperse and assessing connectivity between subpopulations is fundamental for the management and conservation of the species across large wildlife landscapes such as the Kavango Zambezi Transfrontier Conservation Area (KAZA/TFCA), for which wild dogs have been identified as a flagship species.
Recent miniaturization of tracking devices allows researchers to identify and follow dogs and collect information on dispersal movement patterns (e.g., traveled distance) and dispersal success (e.g., survival rate during dispersal) and to evaluate connectivity across the landscapes of KAZA/TFCA. The aim is to improve the long-term viability and connectivity of African wild dog subpopulations.
-
Citizen Science & AI
In addition to scientific research contributions, the computational sustainability community has also contributed technologies that support citizen science conservation initiatives. For example, eBird from the Cornell Lab of Ornithology enables citizens to share sightings of birds and crowd-source the creation of a global bird distribution database for researchers.
-
Social media images, text, and data
Machine learning algorithms can process large-scale data collection from social media images, text, video and metadata, transforming posts into useful inputs.
Professor Brack Hale, based in Iceland’s Westfjords, recently shared in an interview that he uses social media to track people across the landscape, assessing how sensitive tourist locations are to environmental impacts. That information can be used in local infrastructure decisions to answer, “Should we be investing in different structures and interventions to limit environmental impacts?”
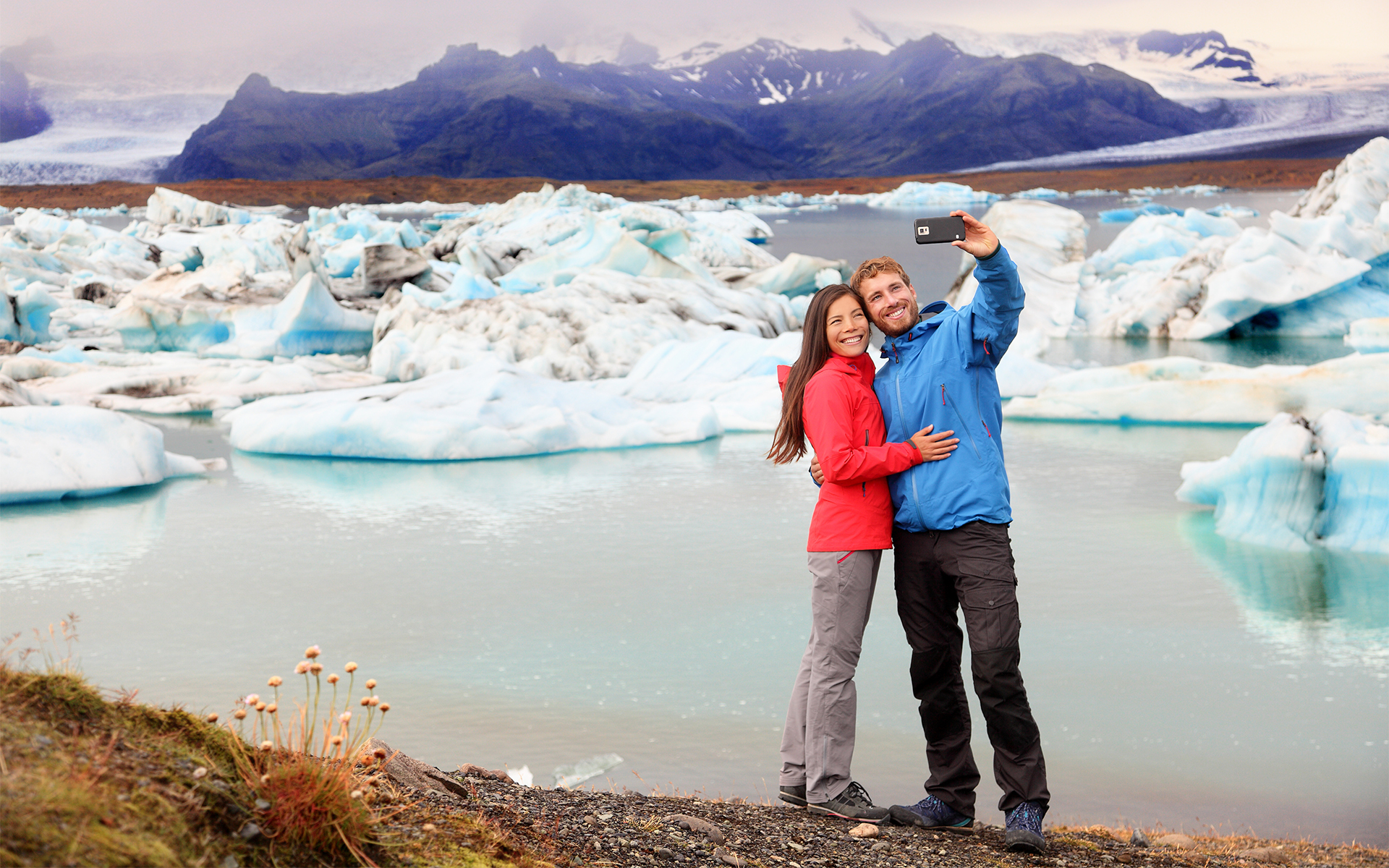
Couple taking a selfie in Iceland
“I was looking at social media content for what kind of plants are in people’s photos. AI models can be used to identify where invasive or non-native species are growing, for example. We can also use them to identify where populations of rare and endangered species are. You have all these people out on the landscape, taking photos regularly. Why not use their eyes to study what’s out there?”
Conservation non-profit Wild Me uses social media to track individual animals in a wildlife population using natural markings, genetic identifiers, and vocalizations and has identified over 50 individual species.
-
Drones
Drones unlock the potential to capture data at finer spatial and temporal resolutions than satellite or airborne remote sensing platforms. Information about a particular environment, individual, or group of organisms can be collected in greater detail with more control. Drone-based data can also be collected more frequently as needed.
Drones are used for a wide variety of conservation purposes, including seed-dropping drones for reforestation, monitoring tigers, rhinos and illegal activities within Nepal’s protected areas, and monitoring and predicting elephant movement in Thailand to reduce human-wildlife conflict.
-
Satellite imagery and data
Satellite platforms offer a broad suite of data of use to scientists, environmental managers and conservation practitioners. Some satellite programs (e.g., Landsat and Copernicus) offer freely available data, whereas commercial operators of higher resolution imagers/sensors (MAXAR, Planet, Airbus) charge for access. Satellite platforms carry a variety of sensors that operate both actively and passively, sensing across the electromagnetic spectrum.
The freely available data archive of the Landsat satellite program makes it a powerful tool for analysis on multi-decadal scales, for example, investigating habitat change in response to storm events over time. The ability to investigate change over such large temporal extents makes satellite-based remote sensing useful for research, management and conservation purposes.
New data-gathering techniques and technologies are increasing the amount of data and also the scale of our perspective on that data. The second development dramatically increasing the use of AI in conservation is increased computing power and the ability to analyze data at scale.
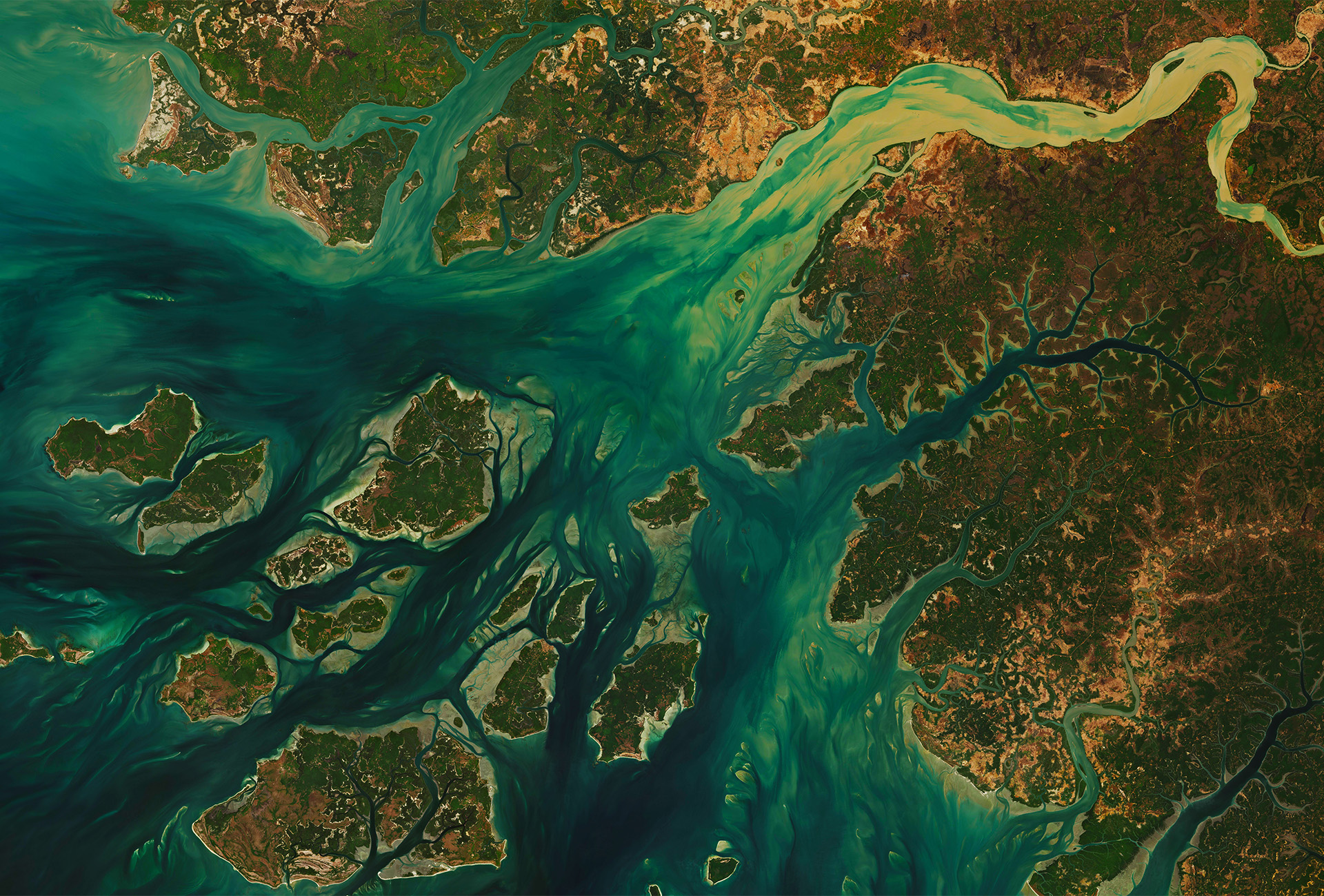
João Vieira-Poilão Islands Marine National Park and the West Coast of Guinea Bissau
In a recent podcast episode from MIT, Dave Thau, WWF’s Global Data and Technology Lead Scientist, explained:
“Changes in machine learning in particular over the past five years even have been enormous. And it goes hand in hand with access to computational resources and data…
In the past, you could do a Ph.D. on one Landsat scene, which is about … 100 kilometers by 100 kilometers… that was, you know, cutting edge. Now, people are typically doing global analysis on these data, accessing millions of these images, and that’s because they’re available and also the computer power is available. What’s going on now is: the speed at which you can do the analyses is increasing and the speed at which the data are collected is also increasing, and that’s all been enabled by this explosion of data and computational power and breakthroughs in machine learning.”
As a result of these changes, there’s wider access to conservation data, and a variety of interested individuals and organizations all over the world can collaborate, building much larger models.
Challenges of Using AI for Conservation
Despite recent advances in AI, the quality of data inputs and the record amounts of data inputs, ambitious efforts to use AI to solve conservation challenges at scale encounter traditional hurdles, like government bureaucracy or a lack of political will or resources.
Other challenges include that the field of computational sustainability and the use of AI for conservation is, in relative terms, quite new, facing a lack of standards and needs to leapfrog or play catch-up so that collaborators can cross-reference from different data sources and streams.
In some parts of the world, there’s a dearth of data scientists. Even when there are many data scientists working on conservation issues, communication can pose a challenge. This is especially true because collaboration and mobilization of resources may require collaboration across a wide variety of interests and perspectives: citizen scientists, local communities, policymakers and scientists.
Finally, when working with AI, there is always concern over data security and privacy, ethics, and data falling to bad actors.
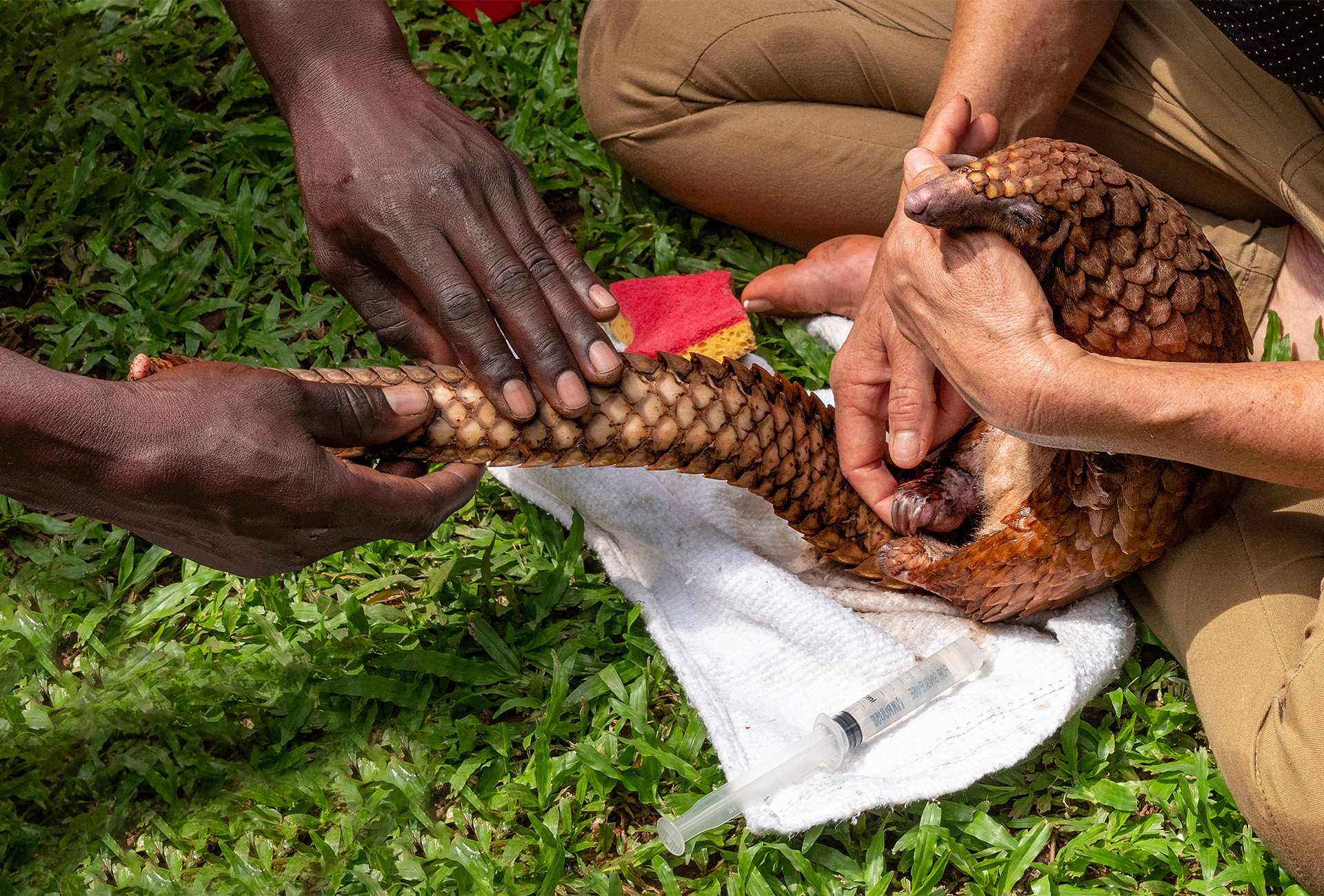
Pangolin rescue in Cameroon. Pangolins are monitored through radio-tracking and wildlife drones. Check out Operation Pangolin on WildLabs.net to learn how the world’s most trafficked mammal is being protected.
AI and the Future of Conservation
In 2022, a supercomputer using AI modeling predicted that around a quarter of all animal and plant species could be extinct by the end of this century. A growing global community of experts and supporters are collaborating to combat species and biodiversity loss.
In this 2019 video on WWF’s work with Google’s Wildlife Insights, Professor Thomas Lovejoy, who coined the phrase biological diversity, said:
I think we’re just at the very beginning of understanding how we can use this very powerful tool, the ability to have our finger on the pulse of these wild places, and then being able to translate that very quickly into advances in conservation for a more sustainable future.
The future of AI in conservation lies in integrating multiple inputs & types of data and building collaborative communities to combat biodiversity and species loss.
If you’re interested in artificial intelligence and conservation or wildlife conservation jobs, check out the 7,600-member conservation technology network at WildLabs.net for events, case studies, articles and more.